I am a Postdoctoral Researcher at University of Pennsylvania, working with Prof. Paris Perdikaris and Prof. Nat Trask. I did my Ph.D. at King Abdullah University of Science and Technology, advised by Prof. Tariq Alkhalifah. I received my Master’s degree from Tongji University, advised by Prof. Yuzhu Liu, and Bachelor’s degree from Jilin university. I also worked as a research intern at Microsoft Research AI4Science and Microsoft Research Asia.
My research interests span the areas of physics-informed machine learning, operator learning, seismic modeling, fluid dynamics, weather modeling, full waveform inversion, deep generative models, and efficient and scalable learning algorithms.
🔥 News
- 2024.10: 🎉🎉 UPenn AIxScience Postdoctoral Fellow!
- 2024.04: 🎉🎉 Two papers published at Neural Networks, and one paper published at IEEE GRSL!
- 2024.03: 🎉🎉 PINN using hash encoding is published at JCP, and another paper is published at GP!
- 2024.02: 🎉🎉 One paper published at IEEE TGRS!
- 2023.05: 🎉🎉 Receive the KAUST PSE Dean’s award!
- 2023.04: 🎉🎉 NeuralStagger is accepted at ICML 2023!
📝 Publications
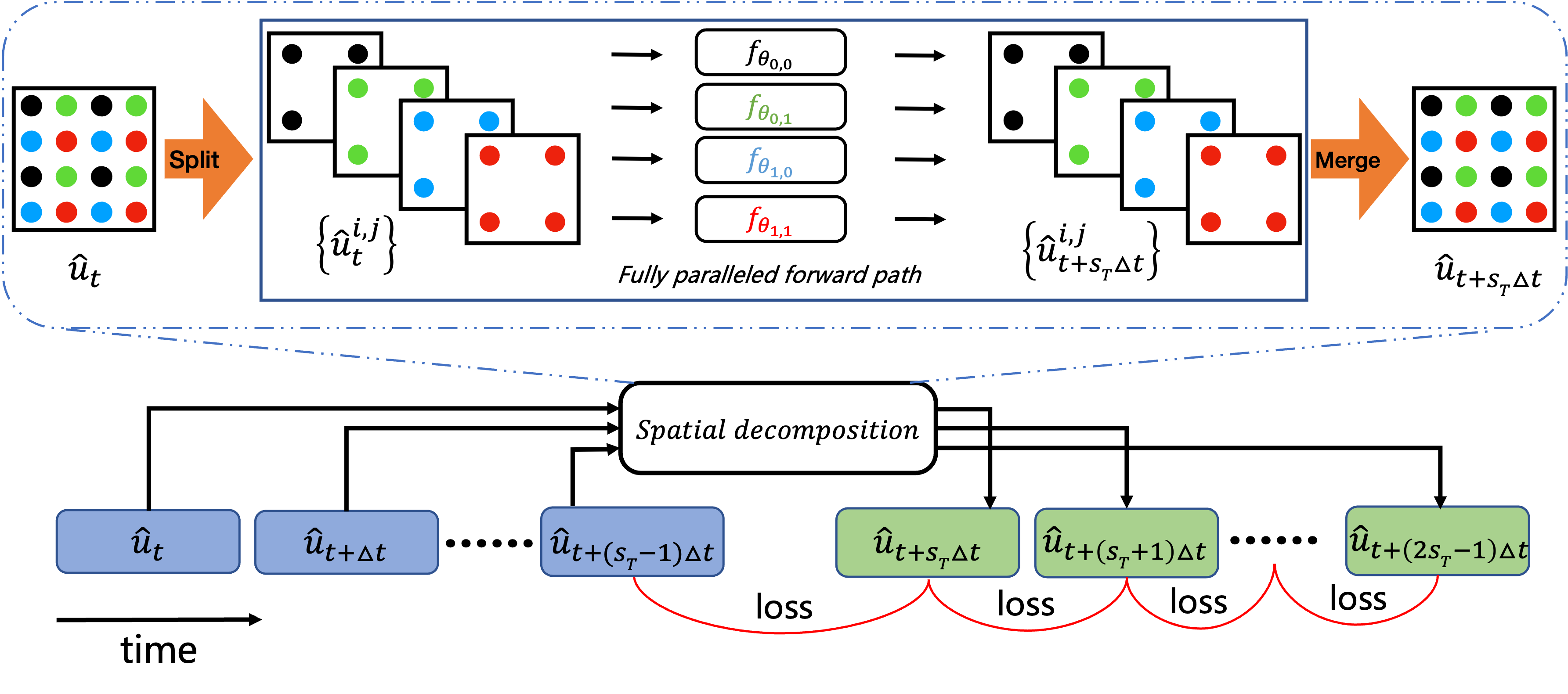
Xinquan Huang, Wenlei Shi, Qi Meng, Yue Wang, Xiaotian Gao, Jia Zhang, Tie-Yan Liu
- A novel, simple, and general spatiotemporal decomposition strategy that speeds up the solution of partial differential equations using neural networks.
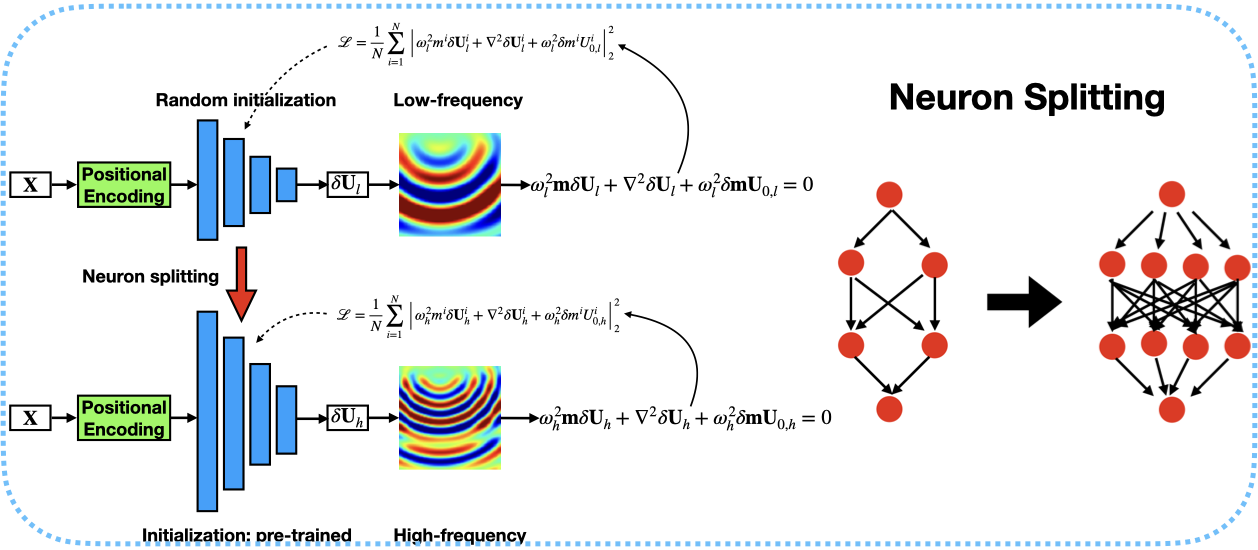
PINNup: Robust neural network wavefield solutions using frequency upscaling and neuron splitting
Xinquan Huang, Tariq Alkhalifah
- A novel physics-informed neural network framework using frequency upscaling and neuron splitting, yielding efficient, highly accurate, high-frequency wavefield solutions.
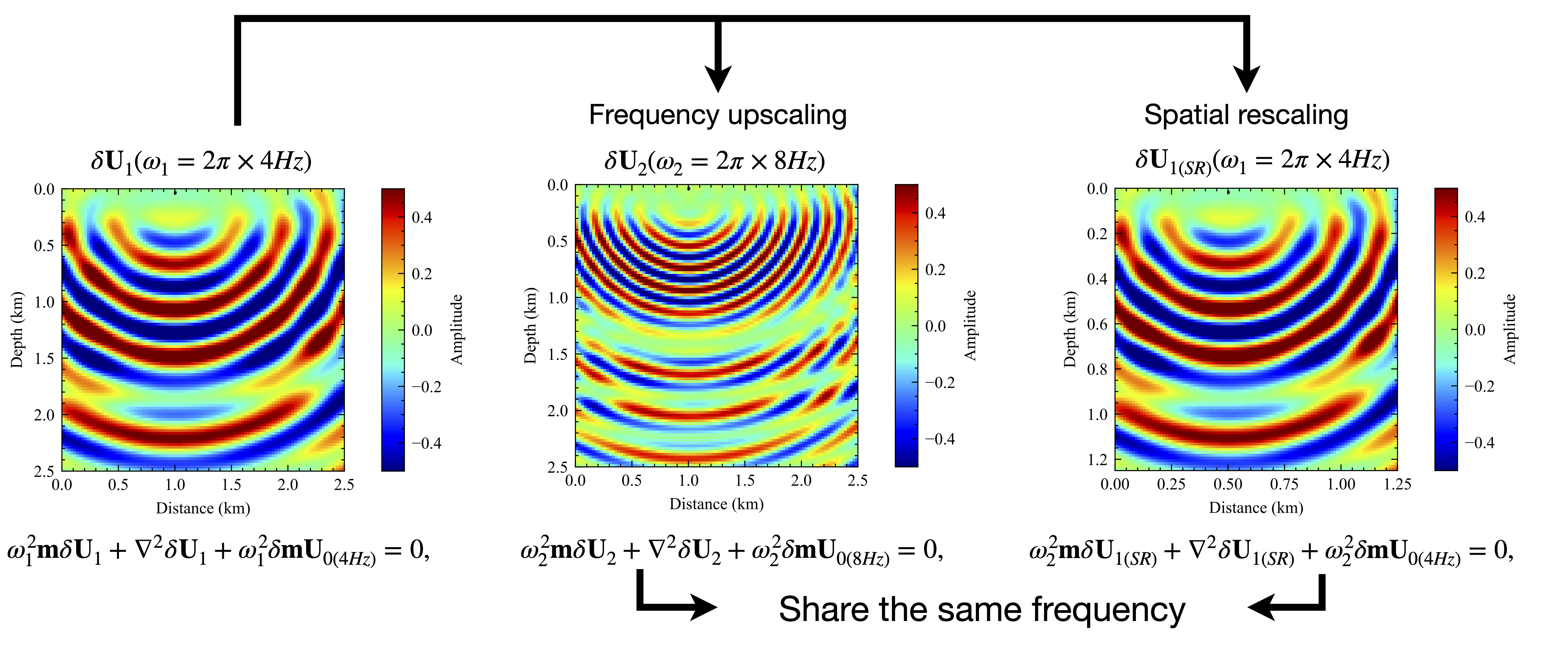
Xinquan Huang, Tariq Alkhalifah
- A Single reference frequency loss function for multifrequency wavefield, which mitigate the change in the spatial wavenumber over frequency by adapting the spatial scale to frequency.
Selected Publications ($\dagger$: Corresponding Author)
JCP
Xinquan Huang and Tariq Alkhalifah, (2024), Efficient physics-informed neural networks using hash encoding, Journal of Computational Physics, 501: 112760.Neural Networks
Xinquan Huang, Wenlei Shi, Xiaotian Gao, Xinran Wei, Jia Zhang, Jiang Bian, Mao Yang, Tie-Yan Liu, (2024), LordNet: an efficient neural network for learning to solve parametric partial differential equations without simulated data, 176: 106354.IEEE TGRS
Xinquan Huang and Tariq Alkhalifah, (2024), Microseismic source imaging using physics-informed neural networks with hard constraints, vol. 62, pp. 1-11, Art no. 4503011.GP
Xinquan Huang and Yuzhu Liu, (2024), An efficient elastic full-waveform inversion of multiple parameters with ocean-bottom seismometer data, Geophysical Prospecting, 72, 2123–2147.Neural Networks
Tariq Alkhalifah and Xinquan Huang, 2024, Physics-informed neural wavefields with Gabor basis functions, Neural Networks, 175: 106286.- Xinquan Huang and Tariq Alkhalifah, 2024, Learned frequency-domain scattered wavefield solutions using neural operators, in revision.
- Xinquan Huang, Fu Wang, Tariq Alkhalifah, 2024, Diffusion-based subsurface multiphysics monitoring and forecasting, under review.
- Xinquan Huang, Tariq Alkhalifah, Fu Wang, 2024, Physics-informed full waveform inversion using learned wavefield solutions, under review.
JGR: Machine Learning and Computation
Fu Wang, Xinquan Huang, Tariq Alkhalifah, 2024, Controllable seismic velocity synthesis using generative diffusion models, 1, e2024JH000153.IEEE GRSL
Mohammad Taufik, Xinquan Huang, Tariq Alkhalifah, 2024, Multiple Wavefield Solutions in Physics-Informed Neural Networks using Latent Representation, vol. 21, pp. 1-5, 2024, Art no. 7504105.ICML 2023
Xinquan Huang, Wenlei Shi, Qi Meng, Yue Wang, Xiaotian Gao, Jia Zhang, Tie-Yan Liu, (2023), NeuralStagger: Accelerating physics constrained neural PDE solver with spatial-temporal decomposition, International Conference on Machine Learning (ICML), 13993-14006.IEEE GRSL
Xinquan Huang and Tariq Alkhalifah, (2023), GaborPINN: Efficient physics informed neural networks using multiplicative filtered networks, IEEE Geoscience and remote sensing letter, vol. 20, pp. 1-5, Art no. 3003405.IEEE TGRS
Fu Wang, Xinquan Huang$\dagger$, Tariq Alkhalifah, 2023, A prior regularized full waveform inversion using generative diffusion models, IEEE Transactions on Geoscience and Remote Sensing, vol. 61, pp. 1-11, Art no. 4509011.JGR: Solid Earth
Xinquan Huang and Tariq Alkhalifah, (2022), PINNup: Robust neural network wavefield solutions using frequency upscaling and neuron splitting, Journal of Geophysical Research: Solid Earth, 127.IEEE GRSL
Xinquan Huang and Tariq Alkhalifah, (2022), Single reference frequency loss for multi-frequency wavefield representation using physics-informed neural networks, IEEE Geoscience and remote sensing letter, vol. 19, pp. 1-5, Art no. 3007105.Geophysics
Yuzhu Liu, Xinquan Huang$\dagger$, Jizhong Yang, Xueyi Liu, Bin Li, Liangguo Dong, Jianhua Geng, Jiubing Cheng, (2021), Multi-parameter model building in the Qiuyue structure with four-component OBS data, Geophysics, 86(5): B291-B301.Patent
Xinquan Huang and Yuzhu Liu, (2020), A source-receiver reciprocal elastic full-waveform inversion for multicomponent OBS data, Patent, patent number: ZL202010499751.8.CJG
Yuzhu Liu, Xinquan Huang, Xianwu Wan, Minao Sun, Liangguo Dong, (2019), Elastic multi-parameter full-waveform inversion for anisotropic media, Chinese Journal of Geophysics(in Chinese), 62(5): 1809-1823.GPP
Yuzhu Liu, Shilin Wu, Weigang Liu, Xinquan Huang, Zheng Wu, (2020), A review of seismic tomographic methods for the inversion of near-surface models, Geophysical Prospecting for Petroleum, 59(1): 1-11.
Conference Papers
EAGE 2024
Xinquan Huang and Tariq Alkhalifah, (2024), Diffusion-based subsurface multiphysics monitoring and forecasting, 85th EAGE Annual Conference and Exhibition, No. 1, pp. 1-5.EAGE 2024
Xinquan Huang and Tariq Alkhalifah, (2024), Learned frequency-domain scattered wavefield solutions using neural operator, 85th EAGE Annual Conference and Exhibition, No. 1, pp. 1-5.EAGE 2024
Fu Wang, Xinquan Huang, Tariq Alkhalifah, (2024), Controllable velocity synthesis using generative diffusion models, 85th EAGE Annual Conference and Exhibition, No. 1, pp. 1-5.EAGE 2024
Mohammad Taufik, Xinquan Huang, Tariq Alkhalifah, (2024), Wavefield Solutions Using a Physics-Informed Neural Network as a Function of Velocity, 85th EAGE Annual Conference and Exhibition, No. 1, pp. 1-5.EAGE 2023
Xinquan Huang and Tariq Alkhalifah, (2023), Microseismic source imaging using physics-informed neural networks with hard constraints: a field application, 84th EAGE Annual Conference and Exhibition, Volume 2023, p.1-5.EAGE 2023
Xinquan Huang and Tariq Alkhalifah, (2023), GaborPINN: Efficient physics informed neural networks using multiplicative filtered networks, 84th EAGE Annual Conference and Exhibition, Volume 2023, p.1-5.EAGE 2023
Fu Wang, Xinquan Huang, Tariq Alkhalifah, (2023), Prior probability regularized FWI using generative diffusion models, 84th EAGE Annual Conference and Exhibition, Volume 2023, p.1-5.SEG 2022
Xinquan Huang and Tariq Alkhalifah, (2022), Source location using physics-informed neural networks with hard constraints, SEG Technical Program Expanded Abstracts: 1770-1774.EAGE 2022
Xinquan Huang, Tariq Alkhalifah, and Fu Wang, (2022), High-dimensional wavefield solutions using physics-informed neural networks with frequency-extension, 83rd EAGE Annual Conference and Exhibition, Volume 2022, p.1-5.ICIP 2022
Tariq Alkhalifah and Xinquan Huang, (2022), Direct imaging using physics-informed neural networks IEEE International Conference on Image Processing, pp. 2781-2785.NeurIPS 2021 workshop
Xinquan Huang and Tariq Alkhalifah, (2021), Single Reference Frequency Loss for Multi-frequency Wavefield Representation using Physics-Informed Neural Networks, NeurIPS 2021 Workshop AI4Science.SEG 2021
Xinquan Huang, Tariq Alkhalifah, Chao Song, (2021), A modified physics-informed neural network with positional encoding, SEG Technical Program Expanded Abstracts: 2480-2484.SEG 2021
Tariq Alkhalifah, Chao Song, and Xinquan Huang, (2021), High-dimensional wavefield solutions based on neural network functions, SEG Technical Program Expanded Abstracts: 2440-2444.SEG 2020
Fu Wang, Huazhong Wang, and Xinquan Huang$\dagger$, (2020), Rotation invariant CNN using scattering transform for seismic facies classification, SEG Technical Program Expanded Abstracts: 1646-1650.SEG 2019
Xinquan Huang, Yuzhu Liu,and Fu Wang, (2019), A robust full waveform inversion using dictionary learning, SEG Technical Program Expanded Abstracts:1506-1510.SEG 2019
Yuzhu Liu and Xinquan Huang $\dagger$, (2019), Full waveform inversion of an OBS dataset acquired from Q field in East China Sea, SEG Technical Program Expanded Abstracts : 1655-1659.
💻 Experience
- 2022.08 - 2023.03, research intern at Microsoft Research AI4Science, Beijing.
- 2022.05 - 2022.08, research intern at Microsoft Research, machine learning Group, Beijing.
📖 Educations
- 2020 - 2024, King Abdullah University of Science and Technology
- 2017 - 2020, Tongji University
- 2013 - 2017, Jilin University
🎖 Honors and Awards
- 2024.10 UPenn AIxScience Postdoctoral Fellowship
- 2023.05 KAUST PSE Dean’s award
- 2021.06 KAUST virtual workshop Lightning talk contest Honorable Mention
- 2020.04 Outstanding graduate student, Tongji University
- 2019.12 Guanghua Scholarship, Tongji University
- 2019.12 Geophysical Scholarship of Tongji University
- 2019.12 Outstanding student paper Annual Meeting of Chinese Geoscience Union (CGU)
- 2019.09 Third prize China Petroleum Society 2019 geophysical exploration technology symposium
- 2018.12 Third prize (Leader) ”HUAWEI Cup” The 15th Post-Graduate Mathematical Contest In Modeling
- 2018.12 Geophysical Scholarship of Tongji University
- 2017.04 National Excellent Project Jilin University ”Undergraduate Innovation and Entrepreneurship Training Program”: Innovation Training
- 2016.08 The Sanhe Aroundwave Software CO. Scholarship, Jilin University First Place Practical Skills Competition of Geophysics, Jilin University
🏫 Teaching
KAUST
- 2023.01 – 2023.05, Teaching Assistant, Advanced Full waveform inversion
- 2021.08 – 2021.12, Teaching Assistant, Seismic Imaging
💬 Invited Talks
- 2024.03, Physics-informed Machine Learning: neural PDE Solvers and their Applications in Subsurface Monitoring, Atmospheric, Earth and Energy Division, Lawrence Livermore National Laboratory
- 2023.10, Accelerating Physics-constrained Neural PDE Solver with Spatial-temporal Decomposition, Purdue University
- 2021.06, Physics-informed neural network for high-frequency wavefield representation, KAUST Virtual Workshop Intelligent illumination of the Earth